Introduction
In this study, a new prediction method, named DAmiRLocGNet, is proposed for human miRNA subcellular localization based on graph convolutional networks (GCNs) and autoencoders (AEs) . DAmiRLocGNet is carried out in five steps: (i) Data Set, collecting data including miRNA subcellular localization information, miRNA-disease associations, disease semantic information, and miRNA sequence information. (ii) Data Preprocessing, giving an overview of the similarity measures used to obtain different similarity network representations from disease semantic information, miRAN-diseases associations, and miRNA sequence. (iii) GCN-based and AE-based feature learning, designing two kinds of feature representation learning from miRNA-disease association information, disease semantic similarity information, and sequence information. GCNs were leveraged to learn miRNA functional similarity latent network representation from miRNA-disease associations network, functional similarity network, and disease semantic similarity network. AE on the miRNA sequence was employed to sequence similarity preserved in low-dimensional representation. (iv) Prediction, splicing the two parts of GCN-based and AE-based feature representation learning representations so as to obtain more comprehensive miRNA functional and sequence feature representations. (v) Result, evaluating and predicting miRNA subcellular localization by evaluation criteria. The flowchart of DAmiRLocGNet is illustrated in Fig.1.
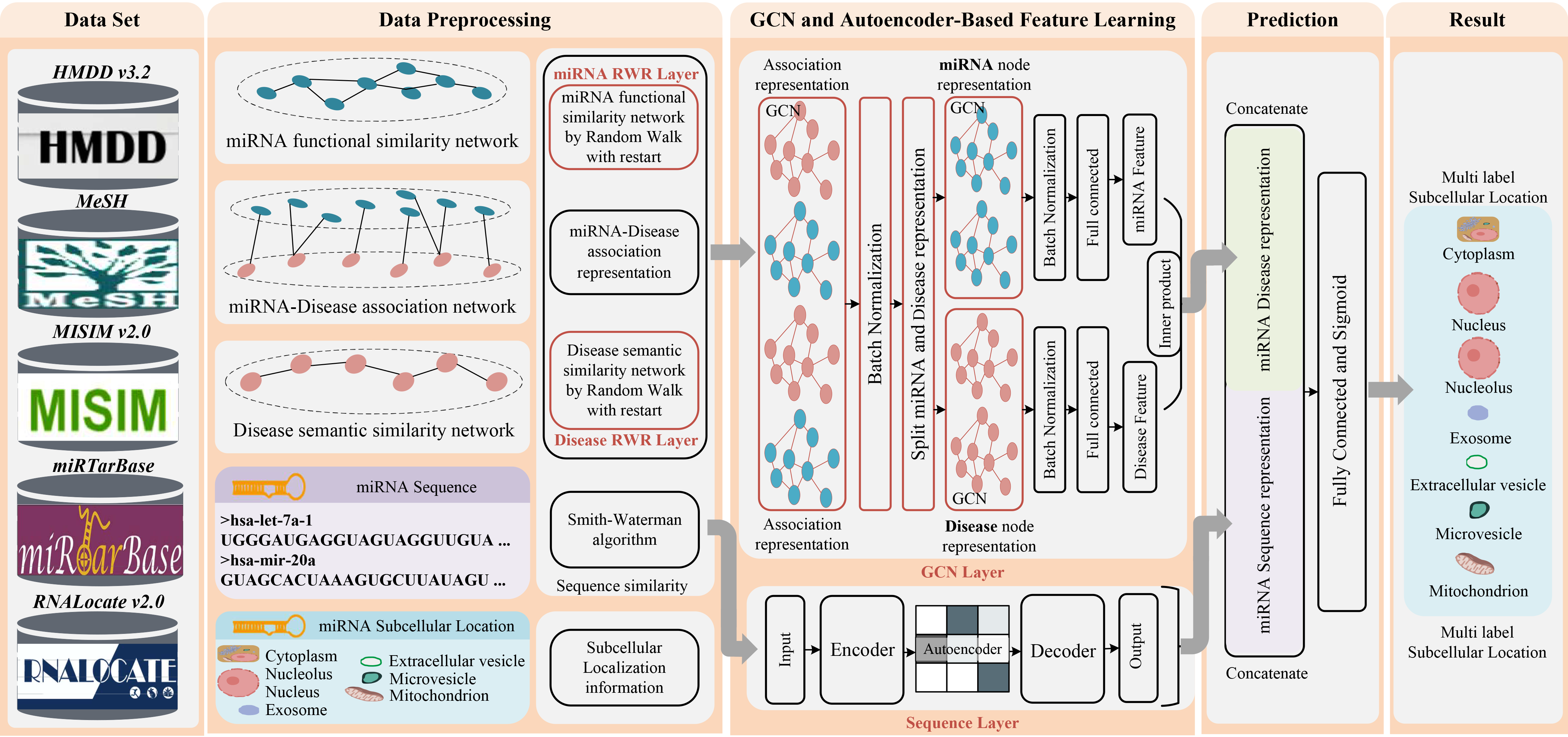
Figure.1 The workflow of DAmiRLocGNet