About FoldRec-C2C
As a key for studying the protein structures, protein fold recognition is playing an important role in predicting the protein structures associated with COVID-19 and other important structures. However, the existing computational predictors only focus on the protein pairwise similarity or the similarity between two groups of proteins from two folds. However, the homology relationship among proteins is in a hierarchical structure. The global protein similarity network will contribute to the performance improvement.
In this study, we proposed a predictor called FoldRec-C2C to globally incorporate the interactions among proteins into the prediction. For the FoldRec-C2C predictor, protein fold recognition problem is treated as an information retrieval task in nature language processing. The initial ranking results were generated by a surprised ranking algorithm Learning to Rank (LTR), and then three re-ranking algorithms were performed on the ranking lists to adjust the results globally based on the protein similarity network, including seq-to-seq model (S2S), seq-to-cluster model (S2C) and cluster-to-cluster model (C2C). When tested on a wildly used and most rigorous benchmark dataset LINDAHL dataset, FoldRec-C2C outperforms other 34 state-of-the-art methods in this field.
The flowchart of FoldRec-C2C is shown in Fig. 1.
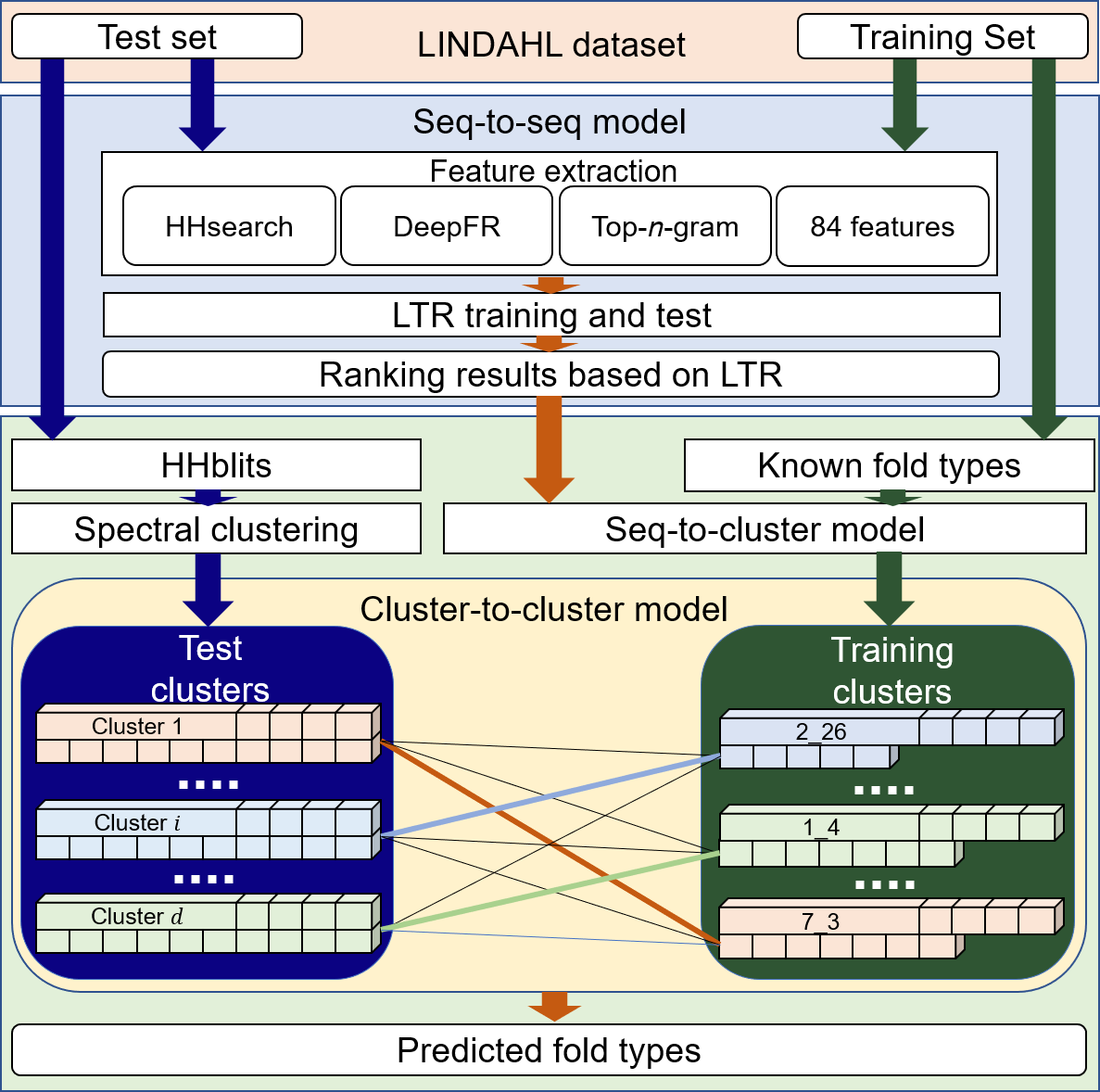