Introduction
Predicting peptide-protein interactions is essential for peptide drug development and protein function research. Recent deep learning-based approaches have shown promising performance, but two challenges remain: how to fully represent and integrate multi-source information such as peptide and protein sequences and structures, and provide actual biological annotation of their interactions, and how to explicitly model and learn residue-level pairwise interactions between peptides and proteins to achieve better predictive performance and interpretability. Here, we propose a knowledge-enhanced interpretable pragmatic analysis method (KEIPA). KEIPA initially integrates multi-source information of peptides and proteins through intra-linguistic contextual representations. It then leverages extra-linguistic contextual representations to construct representation maps of peptide-protein pairs, enabling explicit learning of residue-level pairwise non-covalent interactions. Besides, the knowledge-enhanced module incorporates prior knowledge to promote coordinated interaction between various non-covalent bonds. Finally, using a multi-task learning framework, KEIPA simultaneously predicts peptide-protein non-covalent interactions and their specific non-covalent bond types, enabling biological sequence pragmatic analysis. Experiments on multiple independent test datasets demonstrate that KEIPA achieves superior overall performance compared to state-of-the-art baseline methods and provides interpretable insights into the prediction results. Furthermore, evaluations in other similar biological tasks indicate that KEIPA's method for biological sequence pragmatic analysis is highly generalizable, establishing a new paradigm for AI-driven life science research.
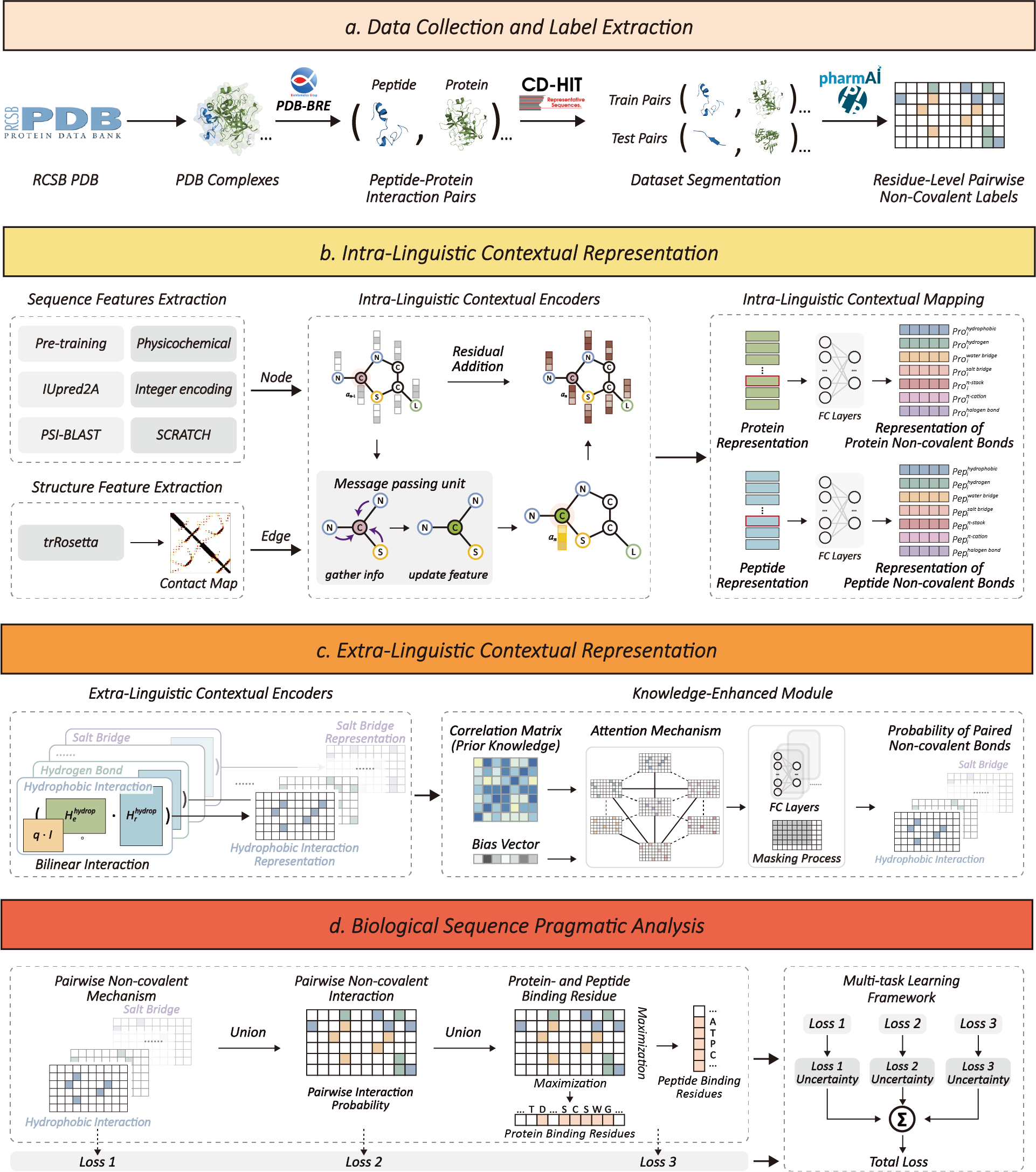
Figure 1. The model architecture of KEIPA. KEIPA is a neural network model designed to achieve biological sequence pragmatic analysis, and it can be mainly divided into two parts: intra-linguistic and extra-linguistic contextual representation.