Introduction
Deep learning-based models for drug response prediction typically face limitations in dynamic environments with ever-evolving data. Continual learning, as a promising approach aiming to acquire new tasks while retaining knowledge from previous ones, is attracting increasing interest. The continual domain shifts caused by the vast drug and genomic spaces and the variability in data acquisition, along with biomedical data privacy regulations, pose significant challenges to current continual learning methods. Moreover, interpretability concept drift and insufficient learning capability in dynamic data environments hinder the effectiveness of continual learning. Here, we propose a novel interpretable multi-contextual self-alignment framework (MCSA) for continual learning in drug response prediction. Specifically, we propose continual self-supervised adversarial learning (CSSAL), which performs multi-domain adversarial attacks to transform current data into adversarial examples of previous tasks and constructs views of current data in old feature spaces, followed by local alignment of representation contexts using multimodal knowledge distillation and the Wasserstein distance. We also design a pluggable pharmacogenomic interpretable module (PPIM) and stable interpretability regularization (SIR) to penalize shifts in features and attention within the old feature space, effectively preventing interpretability concept drift and facilitating biomarker identification. Finally, we develop prototype-aware interactive learning (PAIL), which enhances resource allocation and learning capability through prototype mixture-of-experts and joint fine-tuning. Extensive experiments demonstrate that MCSA outperforms state-of-the-art methods across multiple scenarios and downstream tasks, showcasing its potential to advance reliable drug response prediction and adapt to real-world changes.
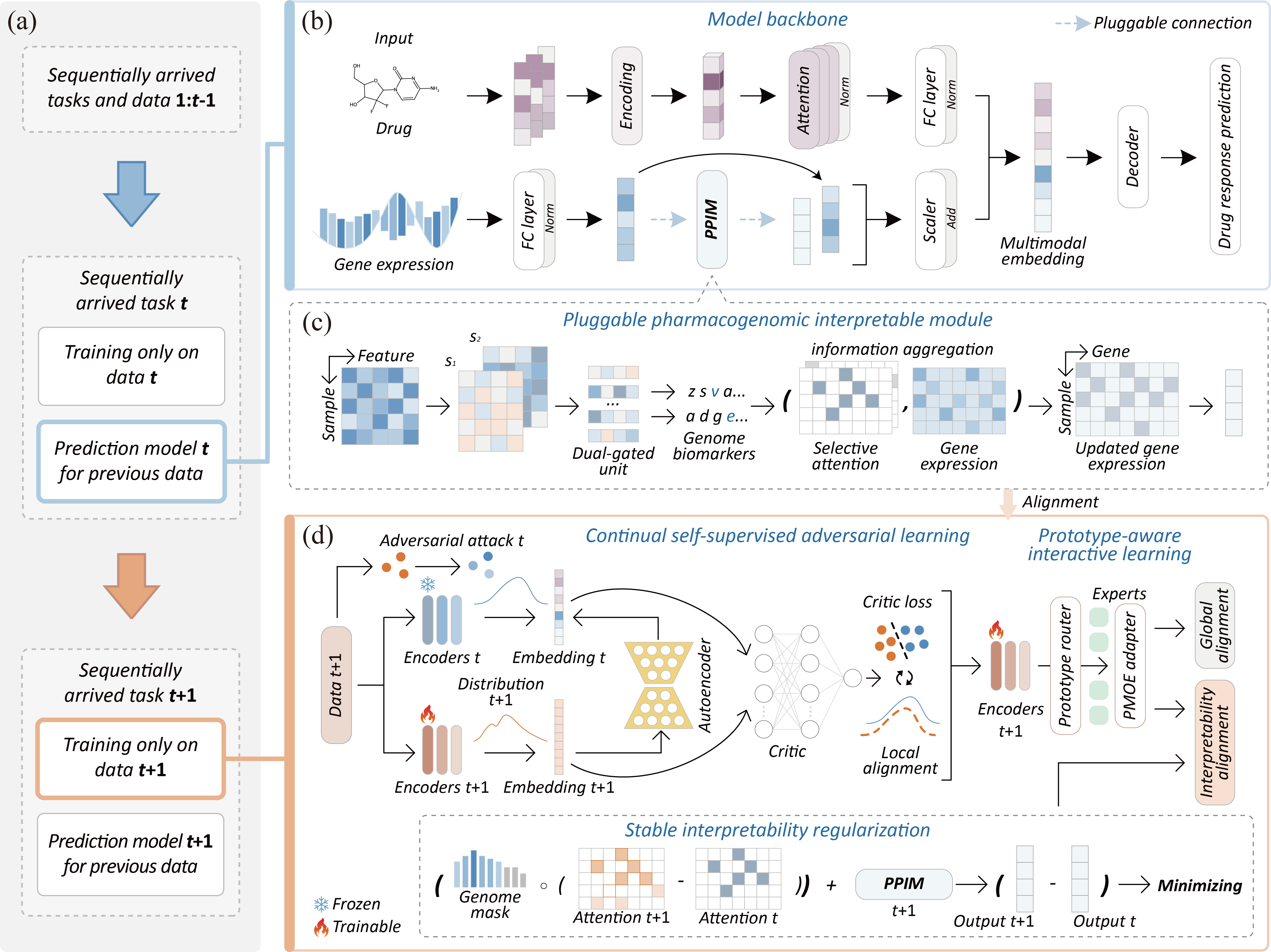
Figure 1. The model architecture of MCSA. MCSA is a continual learning framework designed to adopt changing dynamics in drug response data, and it can be mainly divided into three parts: local alignment, interpretability alignment, and global alignment.