About iDFL-TL
Disordered flexible linkers (DFLs) are functional regions in proteins with high level of flexibility without defined structure. Computational identification of disordered flexible linkers (DFLs) is important for understanding the functions of intrinsically disordered regions (IDRs). Therefore, several computational predictors have been proposed to predict the DFLs only based on the sequence information. However, their performance is limited because of the lack of DFL data (only 140 sequences have been experimentally annotated with DFLs). Different from other IDRs which are able to transition from disorder to order, such as molecular recognition features (MoRFs), DFLs are linkers or spacers between the domains of multi-domain proteins with high level of flexibility without defined structure. DFLs were usually predicted as disordered residues with high confidence by an IDR predictor. Therefore, the features learnt from large IDRs data can be transferred to DFL prediction so as to overcome the data limitation of DFLs.
In this study, we proposed a new predictor called iDFL-TL for predicting the DFLs by combining transfer learning and sequence labelling model derived from the natural language processing (NLP). The sequential labelling model employs Bi-directional Long Short-Term Memory (Bi-LSTM) and Convolutional Neural Network (CNN) to capture the global and local interactions among residues along the whole proteins. Because the DFLs were usually predicted as disordered residues with high confidence by an IDR predictor, the iDFL-TL predictor was pre-trained with large IDR dataset to learn the common characteristics between IDRs and DFLs, and then it was transferred to DFL prediction by fine-tuning with the DFL data to capture the specific features of DFLs. Evaluation on the TE82 independent test dataset showed that iDFL-TL consistently outperforms other exiting predictors with fewer false positives in the ordered regions.
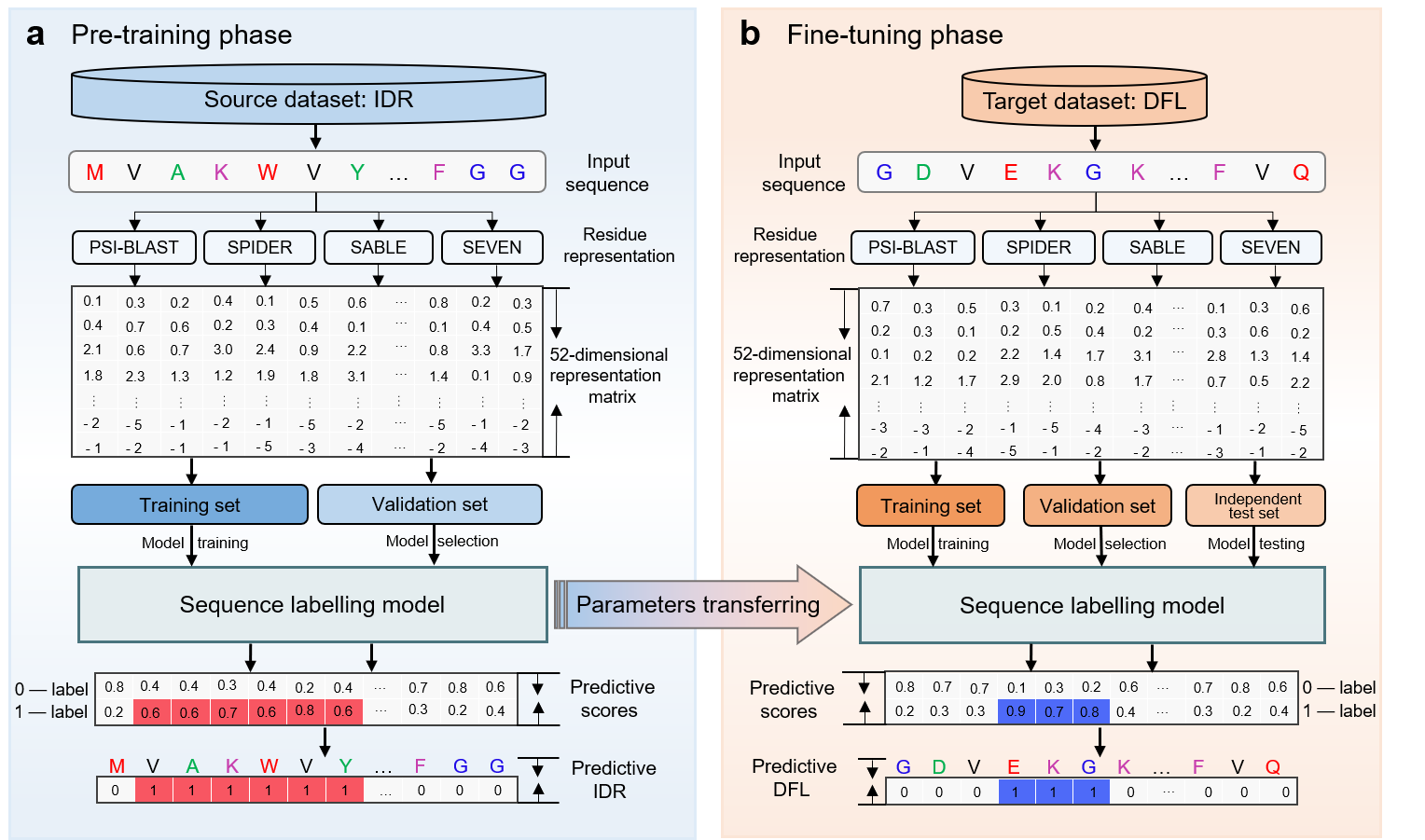